Most data practices are developed around organizational structures: IT owns the data and the data lake itself, while the various line of business data or analytics teams use it. This archaic organizational model hinders entrepreneurial thinking and, subsequently, slows down innovation.
By taking over data ownership altogether, data teams become independent and can bypass red tape completely. A few years ago, this would have been a recipe for disaster, but increased technical literacy, cross-functional teams and the millennials’ need for empowerment enable this decentralized approach to not just function, but lead the organization towards a new area.
Current model is too rigid
As a concept, data lakes are a balancing act. On the one hand, storing everything in every format helps data teams discover and exploit new connections within seemingly unrelated data sets. On the other hand, by dumping everything in a single place one can quickly end up with a heap of undocumented data that nobody owns. That data turns useless as it cannot be relied upon, leading a good idea into a waste of money.
Different organizations have different ideas on how to prevent the lake from becoming, what pundits call, a data swamp:
- Have a staging area for preliminary (raw, unverified, and unprocessed) data and then a “gold” area for curated data, with a strict process of moving between the two stages.
- Have an ingestion filter in front of the lake for data to be cleaned before it is uploaded in the first place. The data lake is all “gold” using the above terminology.
- Use some hybrid mechanism of using the data lake as a staging area and a separate MPP database for “high quality data”.
- Keeping track of all data movement (aka data lineage) so that the original owner, source and transformations of data can all be traced back.
These solutions do their job well in protecting the data lake. In fact, they work way too well, which is why many data lakes are relatively empty. Too much red tape and too many hurdles force timid line of business employees to abandon the idea of looking at some data, because most of the time there is no clear ROI promise to offset the data cleaning effort.
While it is clear that data quality is the primary issue with modern analytics initiatives, forcing more control onto the employees is clearly not the solution.
A trust-based model
The alternative to these quality barriers is moving data ownership to the line of business’s data team and have the members become both users and providers of data to the rest of the organization, as needed.
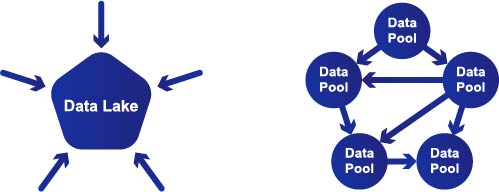
Naturally, when another LOB needs some data, they can simply ask for it, specify data quality requirements, ask for details, and so on. The “maintainer” LOB data team are domain experts and are in the best position to explain what null values mean and can help a “consumer” data team make the most out of that data.
An open culture
Typically, a data team will have their own deadlines and pressure. If there is no other incentive, it’s believed that helping others will fall lower and lower on the priority list. While that might be true for our robot colleagues, the majority of people will want to help others if they can, especially if they’ll need data from them later on.
Knowing that the data you publish is used to train models or that your notebook is useful to others allows data teams to take pride in their work. This is a kind of invaluable incentive that you cannot buy. If the organization has the right values in place and the employees are aligned to those, motivating people to take a break to help someone will be a strength.
The other major reason for keeping data under wraps at IT is security. In a distributed ownership scenario, IT must make sure all parties understand the risks involved and apply the right practices. Most employees understand the repercussions of a data breach. With the proper training, and the proper tools, with definite clearance levels and background checks before granting access to sensitive data, most employees can be trusted with the “family treasure.”
The role of IT moving forward
IT professionals need to fundamentally change from providers of technology, to developers and coaches of best practices for the rest of the organization. Their skill must evolve from deploying tools and technologies on servers, configuring networks, and so on, to training and auditing others. Done right, this is a far more scalable, more efficient and more relaxed environment both for the IT professionals and for the other professionals in the company.